I now
introduce a simple formal model of rugged fitness landscapes, called the
NK model. In this model, N refers to the number of parts of a system –
genes in a genotype, amino acids in a protein, or otherwise. Each part
makes a fitness contribution which depends upon that part and K other
parts among the N. That is, K reflects how richly cross-coupled the
system is. In the geneticist’s term, K measures the richness of the
epistatic interactions among the components of the system.”
Kauffman [1993, 40]
The
problem with any such model is that the ways in which different alleles
at the N loci might be coupled to one another epistatically to produce
an overall fitness for each genotype might be extraordinarily complex.
In general, we truly have almost no idea what those mutual influences on
overall fitness might be. Take Mendel’s peas. He found two alleles for
seed color, yellow and green, and two alleles of a second gene for seed
texture, rough and smooth. A priori we have no idea which of the four
combinations of traits will be the highest fitness, nor how changing
from any one combination of traits to any other will affect fitness. If
the fitness contribution of each gene is epistatically affected by a
large number of other genes, the possible conflicting constraints among
the complex web of epistatically interacting genes are both unknown and
likely to be extremely complex. This complexity suggests that it might
be useful to confess our total ignorance and admit that, for different
genes and those which epistatically affect them, essentially arbitrary
interactions are possible. Then we might attempt to capture the
statistical features of such webs of epistatic interactions by assuming
that the interactions are so complex that we can model the statistical
features of their consequences with a random fitness function. This
leads to the NK model.
Kauffman [1993, 41]
The Kauffman NK model
incorporates each of the three components of the memetic evolutionary
system.
1.
Replications as memes are
transmitted from individual to individual,
2.
Mutation since the copying is
not perfect, and
3.
Selection by the individuals who
carry them for the perceived value they provide to meet that
individual’s wants.
Kauffman begins the
presentation of his NK model by considering the two extreme cases of K =
0 and K = N – 1 followed by the structure of the landscapes that lies
between. The two extreme cases can be examined with relatively straight
forward mathematics. Although the in between situation requires
computer simulation, some reasonable inferences can be made by knowing
the two boundary conditions
K = 0
For K = 0 there are no
interdependencies between the N genes. Each allele at each gene makes a
contribution to the overall fitness function independent of all other
genes. The genotype with all alleles at all genes selected for maximum
fitness is the highest peak in the fitness landscape. Every other point
in the landscape has one or more alleles set to the non-optimal value.
From any point in the landscape the peak can be reached by a series of
one step mutations that will always be to a higher fitness level. Thus
there is only one peak in the fitness landscape.
K = N – 1
The following is a
mixture of direct quotes and paraphrasing of Kauffman [1933, 46 – 60].
I will for the most part spare the reader and myself the tedium of being
precise in my citation. I will not fully repeat his derivations.
The number of local fitness optima is
extremely large.

Where
is
the expected total number of local optima with respect to r-mutant
moves,
a is number of alleles at
each locus, and
N is the number of genes.
The number of expected local optima is

The expected
fraction of fitter one-mutant variants dwindles by ½ at each improvement
step. Starting at any point in the landscape the distribution of
fitter points to the nearest optima is uniform because of the random
fitness function. Thus the average likely next step is halfway to the
peak.
The lengths of
adaptive walks to optima are very short and increase only as a
logarithmic function of N.
,
where r is the length of adaptive walk or number of mutations to
optimum.
The expected time to
reach an optimum is proportional to the dimensionality of the space.
Each step toward optima on average moves half way to the top. Thus
at each step the number of lower fitness mutants doubles. Therefore the
average time to find a new better mutation doubles. Since the number of
steps to reach a local optima is loga((a-1)N-1) the time to
reach the optima is
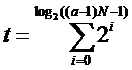
Any genotype can
climb to only a small fraction of the local optima. An estimate is
given by

For a = 20 and
N = 64 there about 1080 optima, but only 1014
can be reached from the lowest ranked genotype.
The converse of this is
also true. Only a small fraction of the genotypes can climb to any
given optimum.
I need to continue this section with complexity
catastrophe and tunable landscapes and especially the conflict of
objectives complexity constraint. And conclude with the point that
memetic systems quickly get trapped and have minimal evolutionary
potential. The mimetic evolutionary system to be presented in a couple
of chapters has far more potential and evolves much faster, however it
requires the existence of the memetic system.
ç
Prior Page of Text
Next Page of Text
è
(C) 2005-2014 Wayne M. Angel.
All rights reserved. |